Researchers learn how to pinpoint malicious drone operators
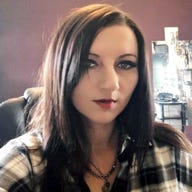
Researchers have come up with a way to pinpoint the location of drone operators seeking to cause harm or disruption in protected airspace.
In 2018, the chaos that can be caused by drone operators near sensitive areas -- such as military bases or airports -- was highlighted by the Gatwick drone incident, in which the UK airport was forced to close for 33 hours between December 19 and 21.
The airport was inundated with passengers attempting to fly, made busier due to the Christmas rush, and had to cancel flight after flight due to a rogue unmanned aerial vehicle (UAV).
In total, 140,000 passengers were impacted by a drone, or several, that were spotted at various locations including runways.
Due to the safety issues linked to drones, including the potential damage of craft, it was not possible for planes to take off -- despite Gatwick's continual efforts to reopen runways during the incident.
A £50,000 ($62,000) reward for any information on the culprit responsible, so far, has led to no leads and no convictions.
See also: A 2-in-1 transformer drone? Coming to a battlefield near you
When a few drones can cause such chaos, being able to pinpoint the location of operators would be a blessing. Now, academics from the Ben-Gurion University of the Negev (BGU) have demonstrated a potential means to do so.
In a research paper (.PDF), "Can the operator of a drone be located by following the drone's path?," published this month, the research team explored how analysis of flight paths may be useful in tracking malicious operators down.
Led by Dr. Gera Weiss from BGU's Department of Computer Science, the team attempted to tackle the problems associated with monitoring flight paths accurately, made more difficult due to the variety of electronic signals all around us.
"Currently, drone operators are located using RF techniques and require sensors around the flight area which can then be triangulated," said researcher Eliyahu Mashhadi. "This is challenging due to the amount of other Wi-Fi, Bluetooth, and IoT signals in the air that obstruct drone signals."
The solution BGU came up with was the use of neutral networking. Rather than focusing on trying to untangle a variety of signals, the network was trained to predict the location of an operator using only flight paths -- even when in motion.
AirSim, an open-source, cross-platform simulator for drones was used to conduct the tests, using 10km of roads and realistic obstacles such as buildings.
CNET: Facebook shared user data with developers after access should have expired
As shown below, the drone would be flown from point A to point B, and a data set containing 81 flights formed the basis of the network's predictive modeling.
In total, the algorithms were able to predict the location of a drone operator with 78% accuracy during simulations, and while the experiment is small, BGU says possible paths towards improvement include improving the machine learning pipeline or even attempts to gain insight into the skill level or training of an operator.
The research team now intends to repeat the experiments with drones in real-time.
TechRepublic: 9 tech products companies can buy for reopening offices during the pandemic
BGU's research was presented at the Fourth International Symposium on Cyber Security, Cryptography and Machine Learning (CSCML 2020) on July 3.
Previous and related coverage
- Best security and surveillance drones for business in 2020: Impossible Aerospace, Microdrones, DJI, and more
- The (not-so-secret recipe) for a commercial drone revolution
- Drones that weave through workers in factories
Have a tip? Get in touch securely via WhatsApp | Signal at +447713 025 499, or over at Keybase: charlie0