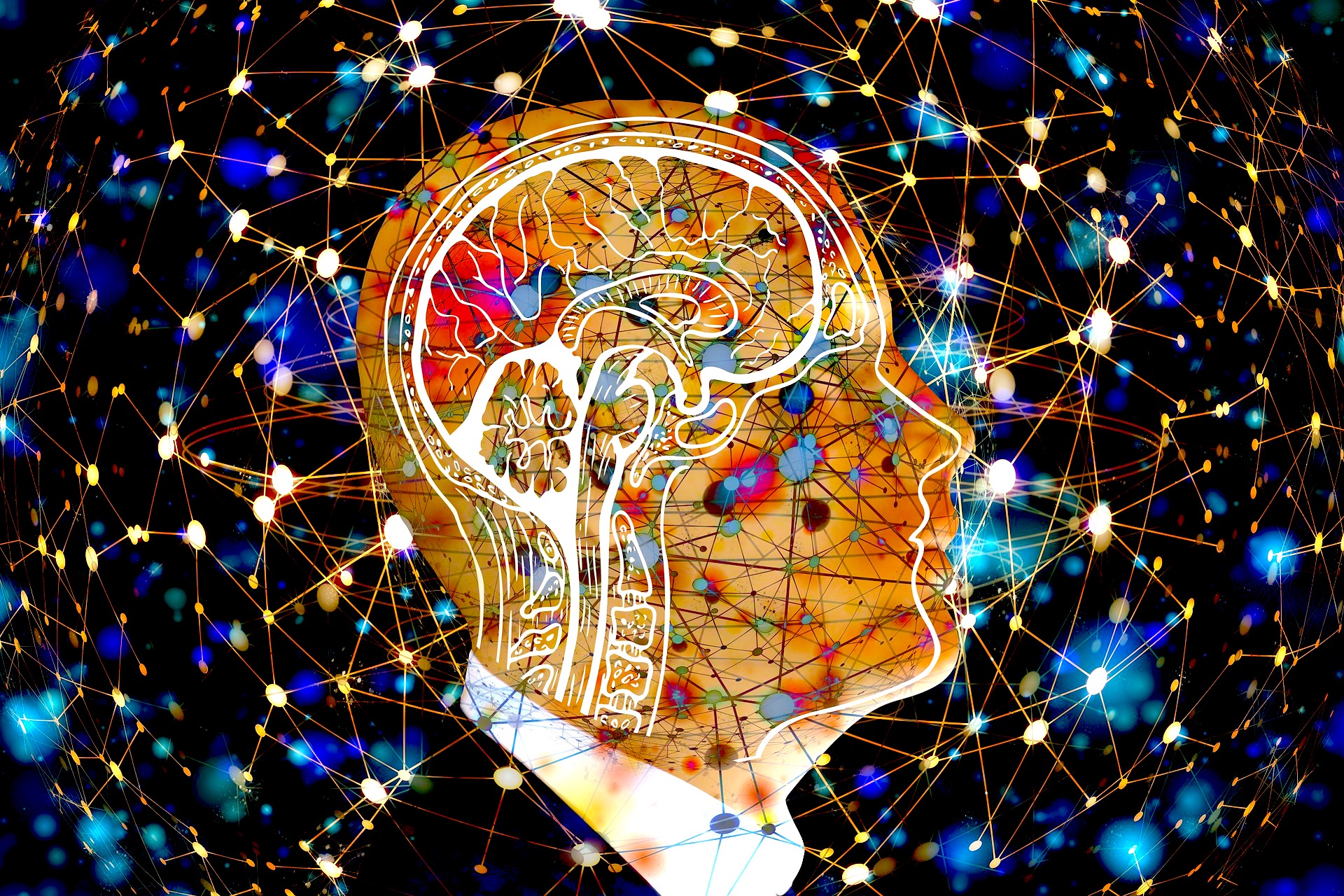
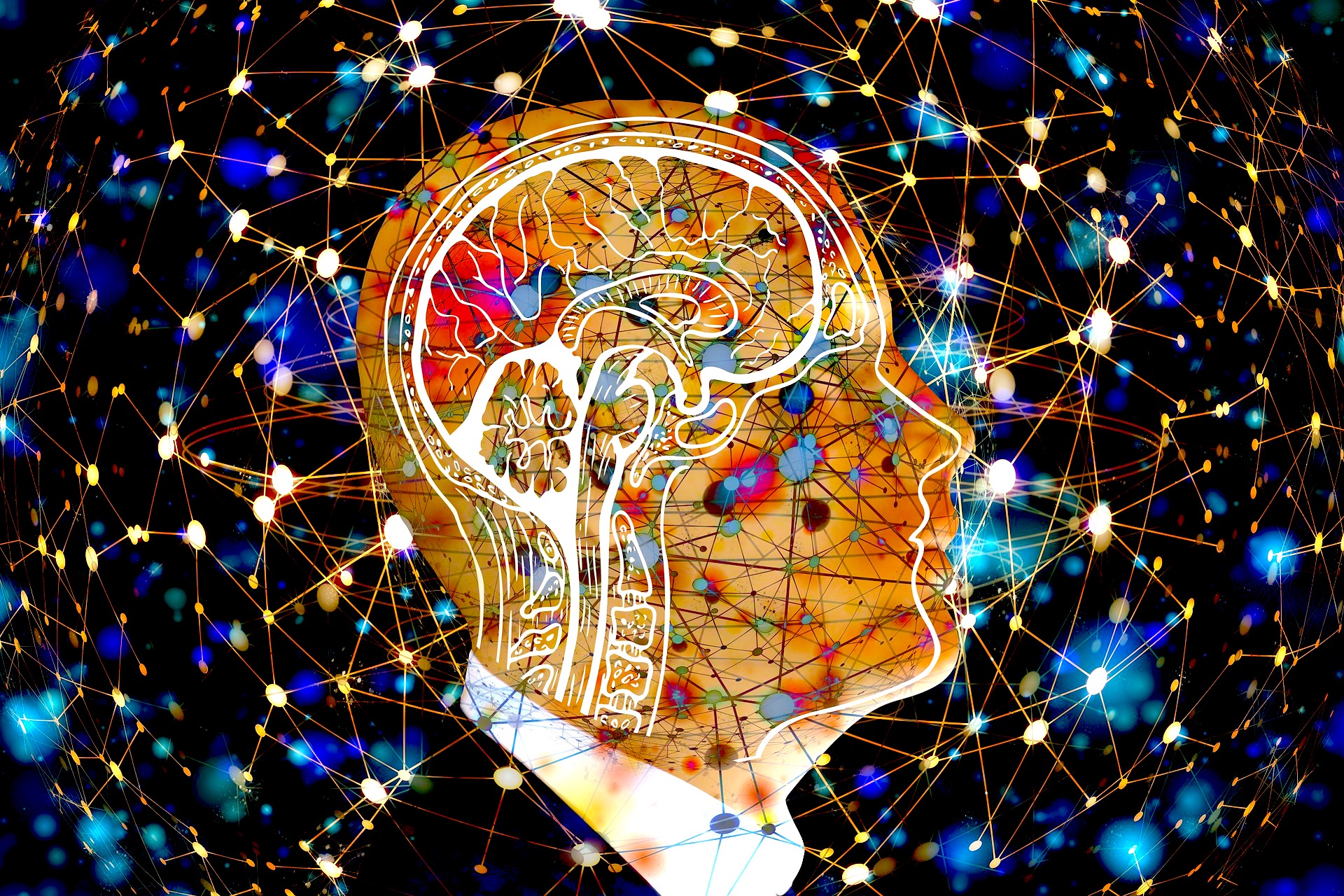
This is the golden age of machine learning. Once considered peripheral, machine learning technology is becoming a core part of businesses around the world. From healthcare to agriculture, fintech to media and entertainment, machine learning holds great promise for industries universally.
Although standing up machine learning projects can seem daunting, ingraining a machine learning-forward mindset within the workplace is critical. In 2018, according to Deloitte Insights State of AI in Enterprise report, 63% of companies invested in machine learning to catch up with their rivals or to narrow their lead. International Data Corp. estimates that by 2021, global spending on AI and other cognitive technologies will exceed $50 billion.
So, the question is no longer whether your company should have a machine learning strategy, but rather, how can your company get its machine learning strategy in motion as quickly and effectively as possible?
Whether your company is just getting started with machine learning, or in the middle of your first implementation, here are the four steps that you should take in order to have a successful journey.
When it comes to adopting machine learning, data is often cited as the No. 1 challenge. In our experience with customers, more than half of the time building machine learning models can be spent in data wrangling, data cleanup and pre-processing stages. If you don’t invest in establishing a strong data strategy, any machine learning talent you hire will be forced to spend a significant proportion of their time dealing with data cleanup and management, instead of inventing new algorithms.
When starting out, the three most important questions to ask are: What data is available today? What data can be made available? And a year from now, what data will we wish we had started collecting today?
In order to determine what data is available today, you’ll need to overcome data hugging, the tendency for teams to guard the data they work with most closely and not share with other groups in the organization. Breaking down silos between teams for a more expansive view of the data landscape is crucial for long-term success. And along the way, you’ll need to make sure you have the right access control and data governance.
On top of that, you’ll need to know what data actually matters as part of your machine learning approach. When you plan your data strategy, think about best ways to store data and invest early in the data processing tools for de-identification and anonymization if needed. For example, Cerner Corp. needed to tackle this challenge to effectively leverage their data for predictive and digital diagnostic insights. Today, the company uses a fully managed service to build, deploy and manage machine learning models at scale.
When evaluating what and how to apply machine learning, you should focus on assessing the problem across three dimensions: data readiness, business impact and machine learning applicability — the chance of success based on your team’s skills.
Balancing speed with business value is key. You should first look for places where you already have a lot of untapped data. Next, evaluate if the area will benefit from machine learning or if you’re fixing something that isn’t actually broken. Avoid picking a problem that’s flashy but has unclear business value, as it will end up becoming a one-off experiment that never sees the light of day.
A good example of solving for the right problems can be seen in Formula One World Championship Ltd. The motorsport company was looking for new ways to deliver race metrics that could change the way fans and teams experience racing, but had more than 65 years of historical race data to sift through. After aligning their technical and domain experts to determine what type of untapped data had the most potential to deliver value for its teams and fans, Formula 1 data scientists then used Amazon SageMaker to train deep learning models on this historical data to extract critical performance statistics, make race predictions and relay engaging insights to their fans into the split-second decisions and strategies adopted by teams and drivers.
Next, in order to move from a few pilots to scaling machine learning, you need to champion a culture of machine learning. Leaders and developers alike should always be thinking about how they can apply machine learning across various business problems.
A common mistake a lot of companies make is putting tech experts on a separate team. By working in a silo, they may end up building machine learning models mostly as proof of concepts, but don’t actually solve real business problems. Instead, businesses need to combine a blend of technical and domain experts to work backwards from the customer problem. Assembling the right group of people also helps eliminate the cultural barrier to adoption with a quicker buy-in from the business.
Similarly, leaders should constantly find ways to make it easier for their developers to apply machine learning. Building the infrastructure to do machine learning at scale is a labor-intensive process that slows down innovation. They should encourage their teams not to focus on the undifferentiated “heavy lifting” portions of building machine learning models. By using tools that cover the entire machine learning workflow to build, train and deploy machine learning models, companies can get to production faster with much less effort and at a lower cost.
For instance, Intuit Inc. wanted to simplify the expense sorting process for their self-employed TurboTax customers to help identify potential deductions. Using Amazon SageMaker for its ExpenseFinder tool, which automatically pulls a year’s worth of bank transactions, Intuit’s machine learning algorithm helps its customers discover $4,300 on average in business expenses. Intuit’s time to build machine learning models also decreased from six months to less than a week.
Finally, to build a successful machine learning culture, you need to focus on developing your team. This includes building the right skills for your engineers and ensuring that your line of business leaders are also getting the training needed to understand machine learning.
Recruiting highly experienced talent in an already limited field is highly competitive and often too expensive, so companies are well-served to develop internal talent as well. You can cultivate your developers’ machine learning skills through robust internal training programs, which also help attract and retain talent.
One approach used by Morningstar Inc., the global financial services firm, used hands-on training for employees with AWS DeepRacer to accelerate the application of machine learning across the company’s investing products, services and processes. More than 445 of Morningstar’s employees are currently involved in the AWS DeepRacer League, which has created an engaging way to upskill and unite its global teams.
If your organization follows these steps, the machine learning culture you build will play a vital role in setting it up for long-term success. There will be growing pains, but at its core, machine learning is experimentation that gets better over time, so your organization must also embrace failures and take a long-term view of what’s possible.
No longer an aspirational technology for fringe use cases, machine learning is making meaningful transformation possible for organizations around the world — and can make a tangible impact on yours too.
Swami Sivasubramanian is vice president of Amazon AI, running AI and machine learning services for Amazon Web Services Inc. He wrote this article for SiliconANGLE.
THANK YOU